
Data Observability: What it is and where to apply it
Data observability enables a more agile and high-performing team, as well as more reliable data and robust workflows.
Have you considered the health of your infrastructure and data tools, and how observability can streamline your processes with them?
Discover how to optimize the performance of your platforms and make more informed and secure decisions.
In this edition, we will show you what observability is, the difference between it and data monitoring, and how having it as an ally can be a differentiator for any company.
Happy reading!
What is Data Observability?
Data observability is related to understanding how your data infrastructure operates and the ability to monitor that operation.
The main idea is to ensure agility in identifying failures in order to analyze and optimize the performance of your infrastructure.
Another important aspect is the ability to make data-driven decisions your infrastructure generates.
This helps maintain data health and drives the resolution of root causes of failures.
How does this work in practice?
Let’s think about your data infrastructure and the (dashboards) where you consume this processed and available data:
- how do you ensure that this data is accurate and reliable?
- how do you guarantee that no information is missing or duplicated?
Data observability ensures this accuracy by monitoring the tests and alerts applied at each stage of your data processing.
Thinking about data observability leads to thinking about data monitoring.
Here’s what distinguishes these two important terms for the field.
Observability, Monitoring, and Data Governance
Observability and data monitoring are fundamental and indispensable tools for data teams.
But you might be wondering: what’s the difference between them and how do they relate?
Monitoring falls under the umbrella of observability, acting more specifically and focusing on the collection of test data.
In turn, observability is more comprehensive.
It appropriates this monitoring data collection to generate insights and make decisions about the behavior of the data structure.
This way, it allows for proactivity in case of a future failure.
Both observability and monitoring are part of data governance, which aims to ensure data quality and security through guidelines, standards, and policies for proper information management.
In summary, even though they may be confusing, the three terms are related in their aim for data quality.
However, they function differently, with governance being at the highest hierarchical level and monitoring at the lowest.
Observe these differences in the image below.

Observability in the Transformation Stage of ETL/ELT
In the data transformation stage, observability applies to observing projects that involve data collection and storage.
This primarily includes monitoring test data, whether successful or not.
Some tools assist in this storage and even create health monitoring dashboards for the structure, as in the following example, which shows Elementary's dashboard. Check it out!
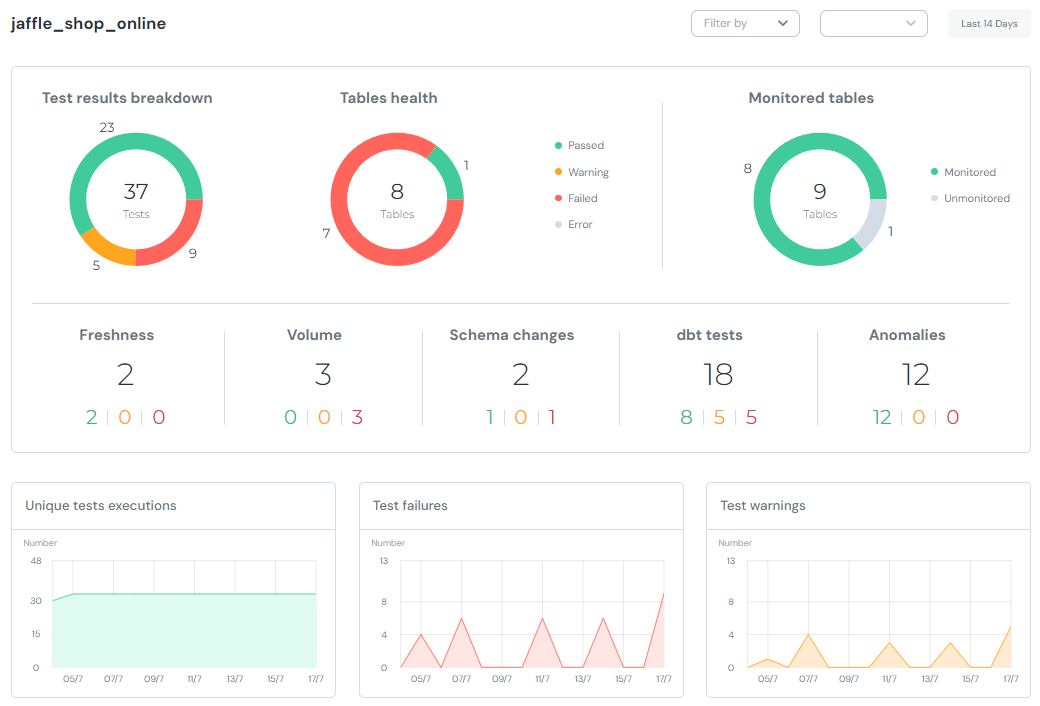
With the storage and monitoring of this data, it is possible to apply Plan, Do, Check, Act (PDCA) routines with planning and analyzes to identify recurring failures and proactively address them, rather than just waiting for failure alerts and then reacting to them.
Next, learn about some tools to prevent this.
Tips on data transformation observability tools
There are several data observability tools focused on analyzing the transformation stages. One tool worth mentioning is Grafana.
Its standout features include:
- Allows the creation of dashboards to visualize and analyze metrics;
- Enables the configuration of alerts based on metrics;
- Can be integrated with other storage tools, such as Prometheus.
If you use dbt in your data transformation stage, it offers tool packages that allow for data observability, such as Elementary Data and Re-Data.
Regarding the functions and qualities of Elementary Data, the highlights are:
- monitors the quality and performance of your data;
- provides direct management within your dbt project;
- controls all test results and alerts, and allows adding anomaly detection tests, which are patterns of suspicious data, to dbt tests;
- stores history of dbt tests and runs, allowing for management based on historical data;
- detects anomalies and dbt tests with failures or schema changes;
- sends alerts to Slack or email;
- calculates predefined and custom metrics;
- integrates directly into your dbt project.
See demonstrations of the monitoring dashboard:
Data Observability: Conclusions
Data observability enables a more agile and high-performing team, as well as more reliable data and robust workflows.
It is revolutionizing the development of data structures in conjunction with monitoring and governance, ensuring greater accuracy, quality, and agility.
Remember: data quality comes from testing and observations.
To continue receiving educational insights like this, subscribe to the Indicium newsletter.
Every 15 days, you'll receive new articles and other updates from the world of data and technology.
Also, take advantage of all the free content available on the website.
See you soon!
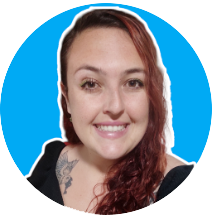
Beatriz Kenup
Analytics Engineer